Using Bayesian Networks in Chemical Risk Assessment: The Role of ChemAlert
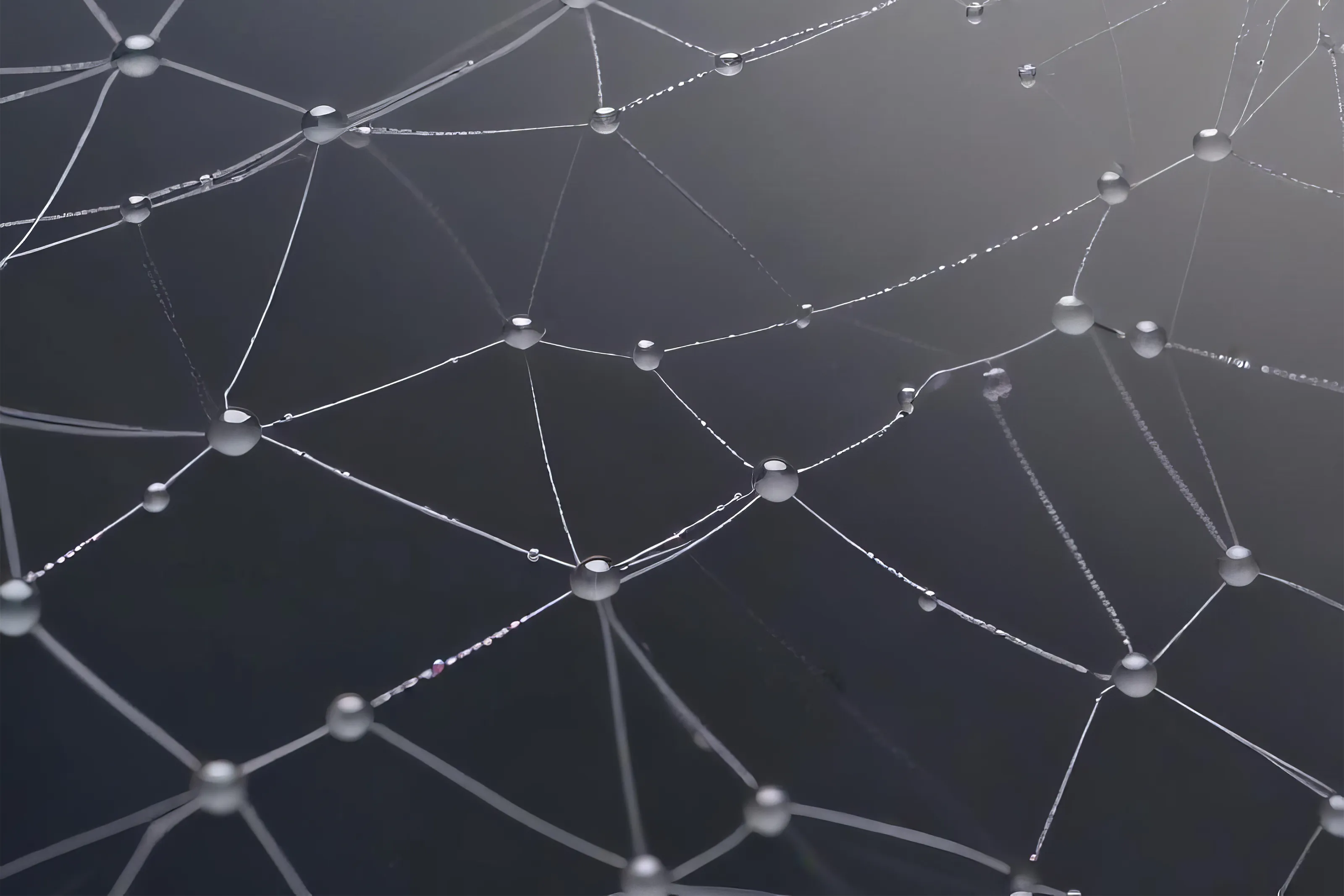
In chemical risk management, understanding the complex interactions between various hazards, exposures, and outcomes is critical for ensuring workplace safety.
Bayesian networks offer a powerful tool for modeling these complexities by providing a probabilistic framework that can help assess, quantify, and manage chemical risks. A Bayesian network is a graphical model that represents a set of variables and their conditional dependencies through a directed acyclic graph (DAG). In the context of chemical risk, it allows risk assessors to model the relationships between chemical properties, exposure levels, toxicity data, and potential adverse health outcomes. This approach provides a structured way to calculate the likelihood of various outcomes based on available data, uncertainties, and expert judgment.
In ChemAlert, Bayesian networks are used to calculate risk scores for chemicals in the workplace, allowing companies to better understand the potential hazards associated with their chemical inventories. By incorporating data on chemical toxicity, exposure routes, regulatory information, and workplace-specific factors (such as duration and frequency of exposure), ChemAlert’s Bayesian network model provides a dynamic risk assessment. It helps users predict the likelihood of adverse health effects like carcinogenicity, mutagenicity, or reproductive toxicity, based on a range of variables. These risk scores are not only quantitative but also probabilistic, taking into account uncertainties in data and offering a more nuanced, realistic picture of risk.
One of the major advantages of using Bayesian networks in chemical risk assessment is their ability to incorporate uncertainty and expert knowledge. In many cases, data on the effects of chemicals may be incomplete, imprecise, or based on extrapolations from animal studies. Bayesian models can integrate this uncertainty into risk predictions, providing a more robust tool for decision-making. For example, if certain data on a chemical’s toxicity is missing, the Bayesian network can still provide a risk score by adjusting for the missing information based on similar chemicals or expert input.
ChemAlert leverages Bayesian networks to help businesses not only assess the risks associated with chemicals in their workplace but also to identify safer alternatives. By comparing risk scores for different chemicals and analyzing the trade-offs between safety and efficacy, companies can make informed decisions about substituting hazardous chemicals with safer alternatives. ChemAlert’s ability to offer a systematic, data-driven approach to risk assessment empowers organizations to prioritize chemicals based on their risk levels and regulatory status, promoting safer workplace environments and compliance with regulations.
In summary, Bayesian networks offer a robust and flexible tool for modeling chemical risks in the workplace, providing a more comprehensive, probabilistic understanding of potential hazards. With ChemAlert, businesses can harness the power of these models to calculate risk scores, manage chemical inventories, and make informed decisions about chemical safety. This helps companies reduce exposure to harmful substances, identify safer alternatives, and comply with safety regulations, ultimately fostering a healthier and safer workplace for all employees.