Machine Learning Meets Chemistry: A Game Changer for Scientific Research
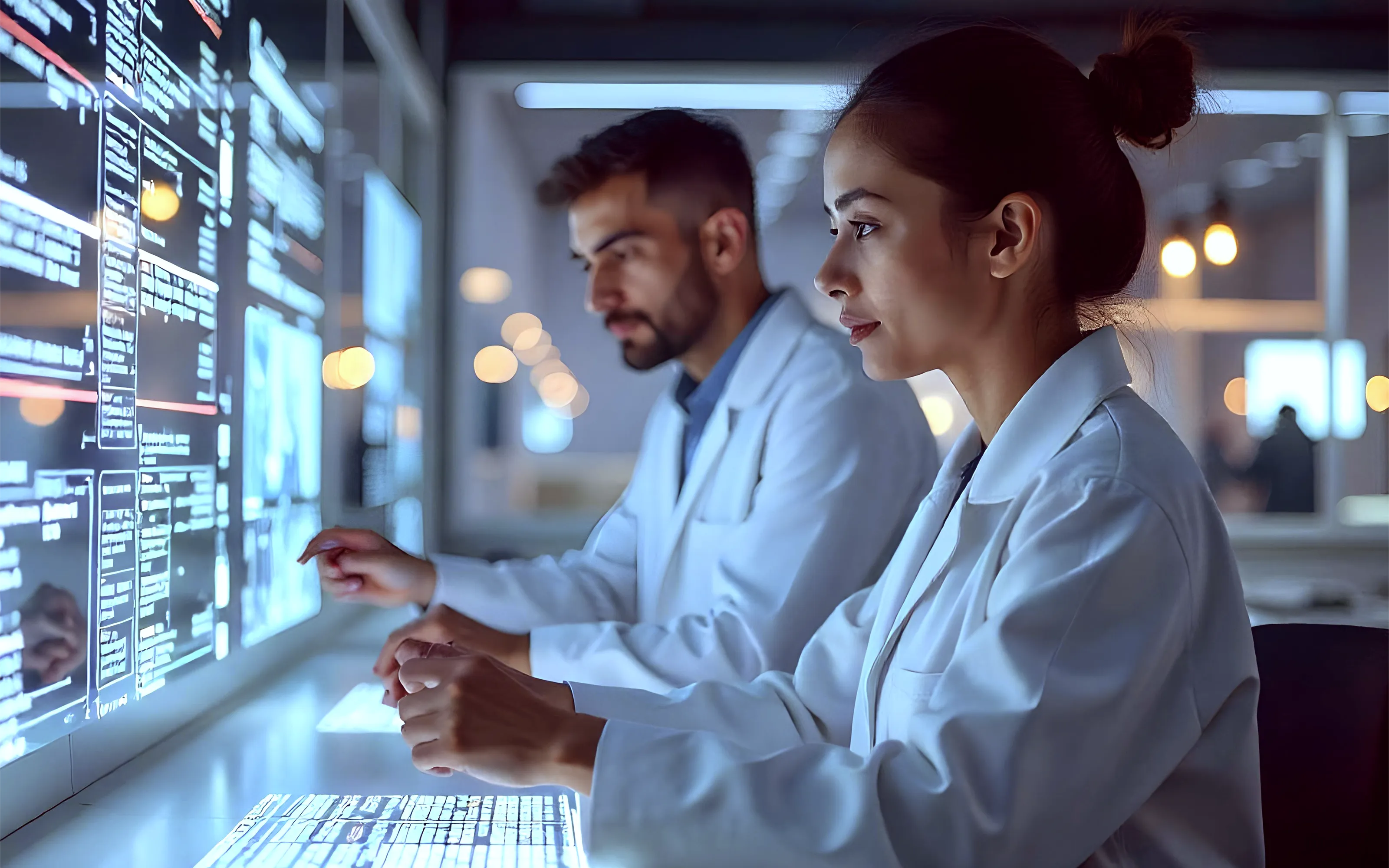
Unlocking Chemistry's Future with GPT-3 and Machine Learning
The fields of chemistry and materials science are undergoing a quiet revolution, thanks to the innovative application of machine learning. Traditionally, work in these fields was hampered by the available amount of relevant data, as well as the need for detailed feature preparation work to represent the domain. However, a new approach is turning the tide—one that leverages large language models trained on vast amounts of text data from the internet.
In a recent article, researchers compared traditional approaches to one using a GPT-3 model fine-tuned to answer a variety of chemical questions. By tailoring GPT-3 for these specific chemical applications, they were able to reach comparable—if not superior—performance to traditional machine learning models, particularly when working with small datasets. This groundbreaking discovery could change how we approach predictive modelling in chemistry and materials science.
Why Does GPT-3 Excel in Chemistry?
One of the greatest challenges in chemistry is the scarcity of large datasets. Traditional machine learning models typically require vast amounts of data to function optimally. However, the fine-tuned GPT-3 was shown to perform better than any other model in the low data regime. Researchers tested the GPT-3 approach on many different tasks, such as predicting molecular properties, chemical reactions, and even materials design, all through natural language queries. GPT-3’s performance on all these tasks rivals existing, more specialized models. Its adaptability to various chemical tasks makes it a versatile tool in the researcher's toolkit.
Transforming the Way We Approach Chemical Research
The most exciting prospect discussed in the paper for using GPT-3 in chemistry is its potential to facilitate "inverse design." This process involves working backwards from a desired outcome, such as a specific material property or reaction yield, to determine the necessary inputs to achieve that result. With their LLM approach, researchers could reverse the questions they asked and still receive accurate predictions, opening up new avenues for exploration.
Implications for the Future of Science
The results of the discussed paper show that there is still untapped potential for foundational LLMs to transform the way science is done. The potential of using them for predictive modelling is another entry into the growing list of their many scientific applications, such as the ability to conduct literature searches, summarise the wealth of collective knowledge, quickly bootstrap projects and explore new research directions.
The potential impact of LLMs in chemistry and materials science is immense. Its ability to democratize machine learning in these fields—making complex models more accessible to a wider range of scientists—is a game changer. In this paper, GPT-3 was used because of the required fine-tuning feature that is not yet available in the state-of-the-art LLMs, such as GPT-4. When this feature is available in those models, another leap in performance is inevitable, unlocking new applications and raw power for scientific inquiry.
To dive deeper into this fascinating study and learn more about the future of AI in chemical research, check out the original research article: https://www.nature.com/articles/s42256-023-00788-1